バックナンバー
当年の月ごとコラム
コラム本文
Coexistence of Artificial intelligence (AI) with academia: a perspective(2024.01)

2024.01.09 Editor W. M.
AI is not a new concept, with the field in academia perhaps originally starting in the mid-1950s. In the decades since, however, AI has moved from the confines of universities and research institutes to the mainstream, and permeates all areas of society. Cycles of groundbreaking research and massive technological evolution have begun to blur the lines between human and machine intelligence. On the one hand, the possibilities for AI to improve many facets of our work and social lives are endless. On the other, AI taps into an age-old human condition: the fear of things that are unknown, or seemingly outside our control. In this column series, we highlight how AI is being used in the academic field, and discuss some of the key issues that researchers—and the wider public—face as we learn to coexist with AI. Before we begin, a question for the readership: how do you know a human has written this introduction?
日本語訳は次へ↓
学術界における人工知能との共存と今後の展望
「人工知能(AI)」という概念が世の中に登場したのは何年も前のことで、学術界では1950年代半ばから取り上げられてきたのではないでしょうか。しかし、学術界で注目を浴び始めてからの数十年間で、AIは大学や研究機関という閉ざされた環境だけでなく、人々の生活のあらゆる場面に取り入れられるようになりました。画期的な研究や目覚ましい技術改革を経た今、人間と機械の知能の境界線は曖昧になり始めています。確かに、AIは仕事の効率や日常生活の利便性を高めるうえで、無限の可能性を秘めています。その一方で、AIは未知の存在であり、制御不能にも見えることから、人間が本能的に恐怖を抱く対象でもあります。今回のコラムシリーズでは、学術界におけるAI活用の現状を紹介しながら、AIとの共存を目指すにあたり、研究者だけでなく一般市民も直面している主な課題を論じます。では、本題に入る前に質問です。「この序章を書いたのは人間だとどうしてわかるのですか?」
Humanities and social sciences(2024.02)

2024.02.02 Editor W. M.
As well as reaching into the future, AI is helping nations rediscover their past. From the cursive kuzushiji texts of Japan to the artistic Fraktur script of Germany, there is a wealth of information and knowledge that to date has been accessible to only a few scholars. AI is now increasing accessibility by recognizing language characters in millions of documents and transcribing it into modern language that can reach a much broader audience. A specific case is kuzushiji, a cursive form of Japanese that is understood by less than 1 in 10,000 of the native Japanese population. A combination of machine and deep learning has empowered computers to first recognize the ancient kuzushiji characters and then convert them into contemporary Japanese. Such work would take hundreds of years for human scholars to complete. The utility of AI in this and similar cases is clear, and few would argue that AI was harmful in this context. However, the automated transcription of texts can pose challenges in the academic sphere. For example, what happens if the majority of original texts portray only one perspective of the society in which they were originally written? Would this lead to a biased perception of that society? Also, who retains ownership of the transcribed data, and how will the transcribed data be used? Academics have a responsibility to ensure that community leaders are fully aware of the scope and aims of their studies, as well as the final destination of the processed data.
日本語訳は次へ↓
人文・社会科学
AI技術は将来を見据えるだけでなく、各国において過去の再発見にも役立っている。日本の「くずし字」からドイツの芸術的なフラクトゥールのような字体に至るまで、これまでは一部の学者しか解読できなかった情報や知識が数多く存在する。現在、AIは文書に含まれる何百万もの文字を認識し、より多くの読者が馴染む現代語へと書き換えて読解のハードルを下げることに貢献している。具体的な例としては、「くずし字」がある。「くずし字」は、日本語を母国語とする1万人に1人以下の割合でしか理解できない、日本語の草書体である。機械学習とディープラーニングを組み合わせることで、古典的な「くずし字」をコンピュータ上でまず認識し、それを日本の現代語に変換することができるようになったが、このような作業は、学者でも膨大な年月を要するだろう。こういった事例や類似した事例でのAIの有用性は明らかであり、この文脈においては有害性を主張する人はほとんどいないだろう。しかし、AIで文書を自動的に書き換えることは、学術的な領域において課題となる可能性がある。例えば、原典の記述の大部分が、当時の社会を一部しか反映していないとしたらどうだろう。当時の社会に対する偏見につながりはしないだろうか。また、書き換えられた文書は誰に所有権があり、どのように利用されるのだろうか。学者には、研究範囲や目的、A Iで処理したデータの最終的な行き先について、コミュニティリーダーが十分理解していることを確認する責任がある。
Natural sciences (Biology)(2024.03)

2024.03.14 Editor W. M.
The Italian polymath Galileo Galilei famously put forward the concept that ‘Mathematics is the language of nature’. How fitting, then, that we should now be using mathematics in the form of AI to understand and interpret natural processes. The sheer complexity of biological processes–––from the molecular and cellular level right up to entire ecosystems––seems a tailor-made challenge for AI to solve. However, AI models need to be trained before they can be set to task. This training requires generation of vast datasets, which in turn is facilitated by advances in technology and experimental methods. Perhaps the most well-known application of AI in the biology field is the use of DeepMind’s AlphaFold suite of programs to predict the structure of proteins. Usually, this requires time- and labor-intensive wetlab experiments such as X-ray crystallography and cryo-electron microscopy. Crystallography remains the gold standard for determination of protein structure. However, AlphaFold can provide an excellent prediction for an average-sized protein in just 15-20 seconds, whereas the lab-based approaches take days. At the other end of the biological scale, AI may help us to conserve precious ecosystems and protect endangered species. For example, the non-profit organization, Imazon, developed the Previsia AI platform to help predict where deforestation of the Amazonian rainforest is most likely to occur. Previsia looks for new roads springing up in the jungle, and then correlates their appearance with multiple other geographical, demographic and environmental parameters. Combined with historical records of deforestation, this allows Previsia to score the likelihood of deforestation in a particular area. In another example, Rainforest Connection have harnessed Arbimon, an ‘acoustic AI’ platform that allows organizations and researchers to listen to the all the sounds within a given ecosystem at scale and over time. This facilitates the identification of novel species, and the tracking of their behavior and response to changing environmental conditions (including those with a negative impact, such as deforestation and poaching). If such approaches can be combined with real-time responses from local and national governments to mitigate the environmental threats, we can look to the future of the natural world with a healthy optimism.
日本語訳は次へ↓
自然科学(生物学)
「自然は数学の言葉を使って書かれている」、イタリアの博学者であるガリレオ・ガリレイがこの概念を提唱したのは有名な話である。そうであるならば、ばAIで数学を自然現象の理解や解釈に利用することはいかにもふさわしい。分子・細胞レベルから生態系全体に至るまで、生物学的プロセスは非常に複雑であるため、AIが解決すべき課題としてはうってつけなのではないだろうか。ただし、AIモデルにこういった課題を課すためには、事前にAIモデルをトレーニングしておく必要がある。トレーニングには膨大なデータセットの作成が必要とされるが、テクノロジーや実験手法の発展によって容易となってきた。生物学分野でもっともよく知られているAIの応用例は、おそらくDeepMind社が開発した、タンパク質の構造を予測するための一連のAlphaFoldプログラムの利用であろう。通常、タンパク質の構造決定には、X線結晶構造解析やクライオ電子顕微鏡など、時間と労力を要するウェットラボの実験が必要である。依然として、結晶学はタンパク質の構造を決定するためのゴールドスタンダードであり、通常、こういった手法では平均的な大きさのタンパク質の構造決定に数日単位の時間を要する。しかしながら、AlphaFoldであれば、わずか15〜20秒で優れた予測結果を得られるのである。他方の生態系規模の話でいうと、貴重な生態系の保全や絶滅危惧種の保護にAIが役立つ可能性がある。たとえば、非営利団体であるImazonは、アマゾン熱帯雨林の伐採が最も起こりそうな場所を予測するために、Previsia AI platformを開発した。Previsiaは、ジャングルの中の新しい道路を探索し、地理的、人口統計的、環境的といった複数のパラメーターを関連付け、森林伐採の過去の記録と組み合わせることで、特定の地域における森林伐採の可能性をスコア化することができる。また別の事例として、Rainforest Connectionは、ある生態系内のすべての音を大規模かつ長期的にわたり、組織や学者が聞くことができる音響AIプラットフォームであるArbimonを活用している。これにより新種生物の同定や、環境条件の変化(森林伐採や密猟など悪影響を及ぼすものを含む)に対する生物の行動や反応の追跡が容易となる。こういったアプローチと、環境上の脅威の軽減を目的とした、地方自治体や国によるリアルタイムの対応を組み合わせることができれば、自然界の未来を健全な楽観主義的に見据えることができるのではないだろうか。
AI and Computer Science(2024.04)

2024.04.19 Editor W. M.
The main goal of research in academic Computer Science is to design and optimize computers and the programs that they run. Studies in this field have real-world benefits including improved cyber security, better healthcare diagnostics and management, and more accurate predictive tools for forecasting financial markets and even the weather! What is the impact of AI in Computer Science? Before addressing this point, we need to take a step back and realize that AI itself is actually a subdiscipline of Computer Science. It can be thought of as one of the tools that Computer Science researchers use to improve the scope, power and efficiency of computing.
So how can AI be used to actually improve research in the field from which it was born? Although this might sound like a paradox, there are some clear examples of where this has been successful. For example, AI can help to improve software code by making it more efficient, or by detecting and fixing bugs. It can also strengthen cybersecurity by looking for vulnerabilities in software that may be susceptible to cyberattacks, and providing protection when an attack is launched. In the examples above, AI is trained on large datasets to recognize issues with pre-existing code. Increasingly, however, students and professionals are turning to AI to actually write code for them from scratch! Here, AI can be given human instructions written or spoken in regular language and convert them into code. Currently, this still requires a degree of human intervention to check the code is actually doing what the user intended. However, improvements in this use of AI could eventually make basic programming accessible to non-experts, and can free up the time of more experienced computer scientists so that they can perform more creative and strategic roles.
日本語訳は次へ↓
AIとコンピュータサイエンス
学術的なコンピューターサイエンスの研究の主な目的は、コンピューターと、コンピューターが実行するプログラムを設計し、最適化することである。この分野の研究は、サイバーセキュリティの向上、より優れた医療診断と管理、金融市場や天候さえも予測するための、より正確な予測ツールなど、実社会で役立つものである!コンピュータサイエンスにおけるAIの影響とは?この点を取り上げる前に、一歩引いて、AI自体が実はコンピューターサイエンスの一分野であることを理解する必要がある。AIは、コンピュータサイエンスの研究者がコンピューティングの範囲、パワー、効率を向上させるために使用するツールのひとつと考えることができる。
では、AIが生まれた分野の研究を実際に向上させるために、AIをどのように使うことができるのだろうか?これはパラドックスのように聞こえるかもしれないが、これが成功した明確な例がいくつかある。例えば、AIはソフトウェアのコードをより効率的にしたり、バグを検出して修正したりすることで、コードの改善に役立てることができる。また、サイバー攻撃を受けやすいソフトウェアの脆弱性を探したり、攻撃が開始されたときに保護を提供したりすることで、サイバーセキュリティを強化することもできる。
上記の例では、AIは既存のコードの問題を認識するために、大規模なデータセットで訓練されている。しかし最近では、学生や専門家がAIに実際にコードをゼロから書いてもらうケースが増えている!ここでAIは、通常の言語で書かれた、あるいは話された人間の指示を与えられ、それをコードに変換することができる。現時点では、コードが実際にユーザーの意図したとおりに実行されているかどうかをチェックするために、人間がある程度介入する必要がある。しかし、このようなAIの使い方が改善されれば、最終的には専門家でなくても基本的なプログラミングにアクセスできるようになり、経験豊富なコンピューター科学者の時間を解放して、より創造的で戦略的な役割を担わせることができるようになるだろう。
AI in Medicine(2024.05)

2024.05.24 Editor W. M.
Artificial intelligence (AI) is rapidly transforming the world of medicine, holding immense potential to revolutionize everything from disease diagnosis and treatment planning to drug discovery and scientific breakthroughs. By analyzing vast amounts of medical data and identifying complex patterns, AI is becoming a powerful tool for healthcare professionals, offering them crucial insights and aiding them in providing better patient outcomes.
AI holds great promise for addressing some key healthcare disparities. For example, many developing countries cannot afford state of the art DNA or RNA sequencing platforms that are required to match cancer patients to optimal treatments. However, images of cancer biopsies can be obtained by staining samples with low-cost reagents. Using many thousands of these images, the hope is that AI algorithms can be trained to identify the features of samples that are associated with specific DNA mutations. Clinicians in developing countries can then match these DNA mutations to specific cancer drugs to customize therapy for individual patients. At the recent American Association for Cancer Research annual meeting in San Diego, AI was prominently featured in many sessions. Translational researchers told us how they are using AI to integrate multiple types of ‘omic’ and clinical data to identify biomarkers that can predict which patients will respond to different drugs. Clinician-scientists explained that AI algorithms can be trained to make diagnoses, or predict treatment outcomes, from ‘looking’ at MRI scans. Companies showcased their generative AI platforms that are capable of generating novel compounds that may one day be advanced into clinical trials. Unlike the temporary darkness during the solar eclipse at the conference, the future looks very bright for AI applications in medicine!
日本語訳は次へ↓
医療におけるAI
人工知能(AI)は医療の世界を急速に変えつつあり、病気の診断や治療計画から創薬や科学の飛躍的進歩に至るまで、あらゆるものに革命をもたらす計り知れない可能性を秘めている。膨大な量の医療データを分析し、複雑なパターンを特定することで、AIは医療専門家にとって強力なツールとなりつつある。
AIは、いくつかの重要な医療格差に対処する上で大きな可能性を秘めている。例えば、多くの発展途上国では、がん患者を最適な治療法に適合させるために必要な最先端のDNAまたはRNAシーケンス・プラットフォームを導入する余裕がない。しかし、がん生検の画像は、サンプルを低コストの試薬で染色することで得ることができる。このような画像を何千枚も使用して、特定のDNA変異に関連するサンプルの特徴を特定するためにAIアルゴリズムを訓練できることが期待されている。そうすれば、発展途上国の臨床医は、これらのDNA変異を特定の抗がん剤と照合し、個々の患者に合わせた治療を行うことができる。
サンディエゴで最近開催された米国癌学会年次総会では、多くのセッションでAIが大きく取り上げられた。トランスレーショナル・リサーチの研究者たちは、AIを使って複数の種類の「オーミック」データと臨床データを統合し、どの患者が異なる薬剤に反応するかを予測できるバイオマーカーを同定していると語った。臨床医科学者は、AIアルゴリズムを訓練することで、MRIスキャンを「見て」診断したり、治療結果を予測したりすることができると説明した。企業は、いつか臨床試験に進む可能性のある新規化合物を生成することができる生成的AIプラットフォームを展示した。学会での日食中の一時的な暗闇とは異なり、医療におけるAI応用の未来は非常に明るいようだ!
The use of AI in streamlining academic processes(2024.06)

2024.06.19 Editor W. M.
In the previous articles, we have taken a look at the role of AI in specific academic disciplines. This final article provides a ‘helicopter view’ of how AI can be used to support processes that are common to research institutes across all disciplines.
Streamlined Recruitment: AI can automate tasks like screening resumes and scheduling interviews for research positions. It can also analyze researcher performance data and publications to identify potential candidates for new projects. Conversely, researchers who are on the job market can make use of some relatively simple AI tools to find prospective employers that match their career goals and personal skillsets.
Grant Writing Assistance and Progress Tracking: AI can analyze successful grant proposals to identify key elements and writing styles. Researchers can leverage this to generate outlines, suggest relevant literature, and even draft initial sections. Additionally, AI-powered dashboards can track progress on active grants, highlighting milestones and upcoming deadlines, ensuring timely completion and compliance.
Demand Forecasting and Inventory Management: AI can also help the purchasing staff at academic institutes in their analysis of past ordering patterns and research project timelines to predict future material needs. This allows for proactive ordering, reducing delays and preventing stockouts. AI can also optimize inventory levels, minimizing storage costs and ensuring researchers have the materials they need when they need them. Additionally, AI can identify frequently reordered items and suggest bulk purchases for cost savings.
Despite these clear benefits, it is important to acknowledge the limitations of AI prior to deploying it in key support areas.
Creativity and Originality: AI struggles with tasks requiring genuine creativity and out-of-the-box thinking. Grant proposals often hinge on novel research ideas and innovative methodologies, which AI can't replace. Human expertise remains crucial for crafting compelling narratives and justifying the significance of the proposed research.
Ethical Considerations: Bias in AI algorithms can lead to unfair hiring practices or skewed grant recommendations. Researchers need to be aware of these limitations and carefully vet AI tools to ensure they are unbiased and ethically sound. Also, when used to assist grant writing, the grant applicant(s) must ensure that they take steps to check and modify AI-generated text and tailor it to their own research prior to submission. Otherwise they run the risk of being labelled plagiarists. Ultimately, AI should be seen as a valuable tool to empower human researchers, not replace them. By leveraging the strengths of AI for administrative tasks and initial guidance, researchers can free up time and mental space to focus on the core aspects of research: conceptualizing groundbreaking ideas and conducting rigorous investigations.
日本語訳は次へ↓
学術プロセスの合理化におけるAIの活用
これまでの記事では、特定の学術分野におけるAIの役割を見てきた。最終回となる本稿では、あらゆる分野の研究機関に共通するプロセスをサポートするためにAIをどのように活用できるかについて、「ヘリコプターの視点」で解説する。
採用活動の合理化: AIは、研究職の履歴書のスクリーニングや面接の日程調整といった作業を自動化することができる。また、研究者の業績データや論文を分析し、新規プロジェクトの候補者を特定することもできる。逆に、就職活動中の研究者は、比較的シンプルなAIツールを活用することで、自分のキャリア目標や個人的なスキルセットにマッチする就職希望先を見つけることができる。
助成金申請の支援と進捗管理: AIは、成功した助成金提案書を分析し、重要な要素や書き方を特定することができる。研究者はこれを活用して、アウトラインを作成し、関連文献を提案し、最初のセクションの草稿を作成することもできる。さらに、AIを活用したダッシュボードは、アクティブな助成金の進捗状況を追跡し、マイルストーンや今後の期限を強調表示することで、タイムリーな完了とコンプライアンスを保証します。
需要予測と在庫管理: AIは、学術機関の購買スタッフが過去の発注パターンと研究プロジェクトのスケジュールを分析し、将来の材料ニーズを予測する際にも役立ちます。これにより、先手を打った発注が可能になり、発注の遅れを減らし、在庫切れを防ぐことができる。また、AIは在庫レベルを最適化し、保管コストを最小限に抑え、研究者が必要な時に必要な材料を確保することができる。さらに、AIは頻繁に再注文される品目を特定し、コスト削減のための大量購入を提案することもできる。
このような明確な利点があるにもかかわらず、重要なサポート分野にAIを導入する前に、AIの限界を認識することが重要である。
創造性と独創性: AIは、真の創造性と既成概念にとらわれない思考を必要とするタスクに苦戦する。助成金の提案では、斬新な研究アイデアや革新的な方法論が鍵となることが多いが、これはAIでは代替できない。説得力のある物語を作り上げ、提案された研究の重要性を正当化するには、人間の専門知識が不可欠であることに変わりはない。
倫理的配慮: AIアルゴリズムのバイアスは、不公正な雇用慣行や偏った助成金推薦につながる可能性がある。研究者はこのような限界を認識し、AIツールが公平で倫理的に健全であることを確認するため、慎重に吟味する必要がある。また、助成金申請者は、助成金申請の補助に使用する場合、提出前にAIが生成した文章を確認・修正し、自身の研究に合うように調整する手順を確実に踏まなければならない。そうしないと、盗作者というレッテルを貼られる危険性があるからだ。
結局のところ、AIは人間の研究者に取って代わるものではなく、人間の研究者に力を与える貴重なツールと見なされるべきである。管理業務や初期指導にAIの長所を活用することで、研究者は時間と精神的余裕を確保し、研究の核心部分である画期的なアイデアの構想や厳密な調査に集中することができる。
2024年上半期連載コラム [学問とAI] の種明かし(2024.07)
2024.07.11 コーディネーター
今年の1月から半年間、続きました校閲者W.Mの連載コラム、実は途中から日本語訳をAIが担当しておりました。
皆様は気付きましたか?
W.Mもコラム内で申しております通り、AIだけの力では未だ及ばぬところも多々あります。AIの翻訳も素晴らしいものでしたが、では、人間の翻訳はいかがなものか?ぜひ、弊社の翻訳者A.Nによる最終章の翻訳をご覧ください。
今後もご依頼時にAIx人力翻訳とするか、人力のみの翻訳とするか、ご検討時のサンプルとしていただけますと幸いでございます。
================
学術プロセスの合理化におけるAIの活用
以前の記事では、特定の学術分野においてAIが果たす役割についてみてきました。最終回となる今回は、あらゆる分野の研究機関に共通する学術プロセスのサポートに対してAIがどのように活用できるか、その可能性について概説したいと思います。
採用活動の合理化:AIの活用により、研究職ポジションの人材を募集する際の履歴書の選別や面接の日程調整といった作業を自動化することができますし、また研究者の業績データや論文を分析することで、新規プロジェクトの候補者選定も可能です。立場を変えると、就職活動中の研究者は、比較的簡便なAIツールを活用することで、自身のキャリア目標や研究者自身のスキルセット(スキル、能力、経験)に合致する雇用候補を見つけることができます。
研究費申請書の作成支援と進捗管理: AIは過去に研究費獲得に成功した申請書を分析し、重要なポイントや書き方を抽出することができます。研究者はこれらを活用して、アウトラインの作成、関連した文献の提案、第一段階の草案作成まで行うことができます。さらに、AIを搭載したデジタルダッシュボードは、進行中の研究課題の進捗状況を追跡し、マイルストーンや以降の期限を強調して表示することで、適時遂行と期限遵守を確実にします。
需要予測と在庫管理:過去の発注パターンや研究プロジェクトのスケジュールをAIで分析することにより、研究機関の購入担当者が将来の物品需要を予測する際に役立ちます。これにより先手を打った発注が可能になり、発注の遅れを減らし、在庫切れを防ぐことができます。また、AIは在庫量を最適化し、保管費用を最小限に抑え、研究者が必要な時に必要な材料を確保することができます。さらに、AIは頻繁に再注文される品目を特定して大量購入を提案することで、コスト削減も可能になります。
このようにAIには明確な利点がありますが、重要なサポート分野に対してはAIを導入する前にその限界を認識しなければなりません。
創造性と独創性: AIにとって、真の創造性や既成概念にとらわれない思考を必要とする仕事は苦手と言えます。多くの場合、研究費申請では斬新な研究アイデアや革新的な方法論が鍵となりますが、この点はAIでは代替できないものでしょう。説得力のあるストーリーを作り上げ、申請研究の意義を納得させるためには、人間の専門知識が不可欠であることに変わりはありません。
倫理的配慮: 万が一、AIのアルゴリズムに偏りがあると、不公平な雇用慣行や偏った研究費審査につながる可能性があります。こういったAIがもつ限界を研究者は認識した上で、AIツールが公正中立、かつ倫理にかなっていることを慎重に吟味する必要があります。また、研究費申請者が申請の補助に使用する場合は、提出前にAIが生成した文章を確認、修正し、自身の研究に合うように調整する手順を確実に踏まなければなりません。そうでなければ、盗作者というレッテルを貼られる危険性があります。
結局のところ、AIは人間の研究者に取って代わるものではなく、あくまでも研究者に役立つ貴重なツールと見なされるべきです。管理業務や初期指導にAIの長所を活用することで、研究者は時間と精神的余裕を確保し、研究の核心部分である画期的なアイデアの着想や緻密な調査に集中して取り組むことができるのです。
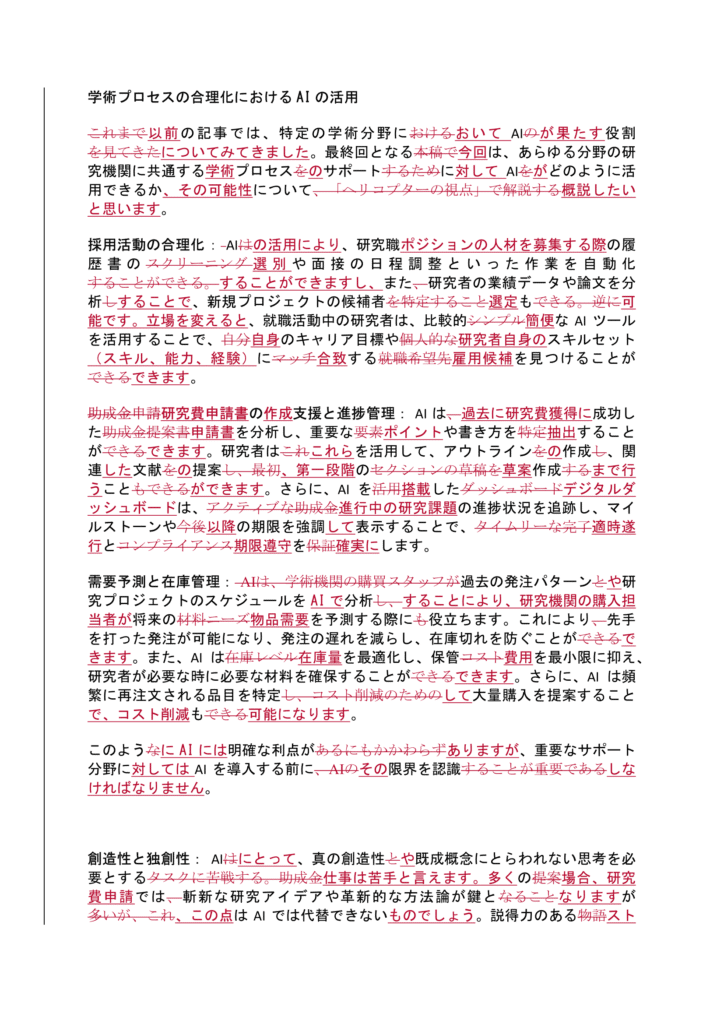
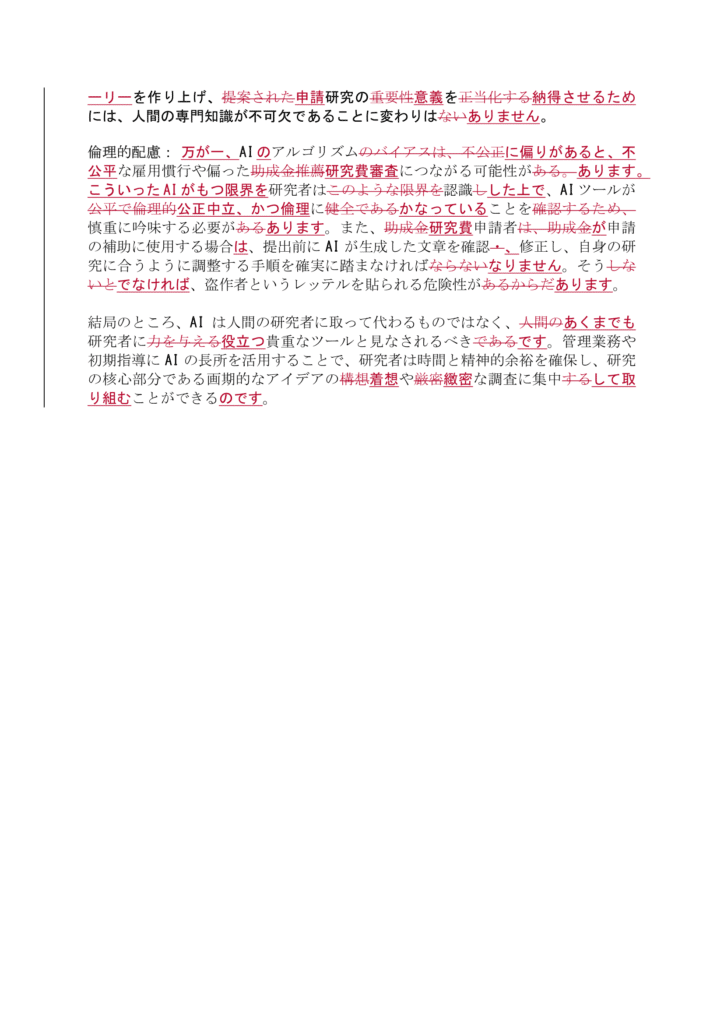
Predatory Journals: An Escalating Threat to Academic Credibility(2024.08)

2024.08.06 Editor T. J.
Have you ever received an unsolicited request from a journal desperately asking you to submit a manuscript on any topic, promising fast publication? If it sounds too good to be true, it usually is! Indeed, if you think about it, we know that publishing in reputable journals is a time-consuming and competitive process, so why would a genuine journal be searching for papers? The truth is that these requests often come from predatory journals seeking your money, not your scientific excellence.
Predatory journals exploit gaps in institutional policies and the pressure on academics to publish frequently. They use deceptive practices such as mimicking the names of reputable journals and listing scholars as board members without their consent. And their approaches are becoming more and more sophisticated, making them harder to distinguish from legitimate ones. They often feature professional-looking websites and conduct superficial peer reviews to mimic credibility. This misleads researchers, especially those from resource-limited regions, into submitting their work to these outlets. As such, identifying a predatory journal requires a keen eye for the tell-tail signs such as typographical errors, obscure contact email addresses, unbelievable publication times, high acceptance rates, and high upfront fees.
The erosion of academic integrity is a significant issue arising from predatory journals. Some scholars knowingly publish in them due to shorter review times and lower rejection rates compared to reputable journals, leading to the propagation of poor-quality research. Thankfully, institutions are starting to combat the influence of predatory journals by enhancing support for researchers and emphasizing the importance of publishing in credible journals. In summary, while predatory journals are becoming more sophisticated, increased awareness and institutional support are essential to safeguard the integrity of scientific research and publication.
日本語訳は次へ↓
ハゲタカジャーナル: 高まる学術的信頼性への脅威
ジャーナルから、どのようなテーマでも構わないから原稿を投稿して欲しいと熱心に依頼され、早期の出版を約束されたことはないでしょうか?もし、それが余りにも出来すぎた話だと感じたら、たいていはその通りでしょう!確かに、よく考えてみれば、評判の高いジャーナルに論文を掲載するのは、時間がかかる競争的なプロセスが必要であることはわかっている。このような依頼は、あなたの科学的卓越性ではなく、あなたのお金を求める略奪的ジャーナルから来ることが多いということが真実なのです。
ハゲタカジャーナルは、研究機関の方針のズレや 頻繁に論文を出版しなければならないという研究者へのプレッシャーを悪用します。また、評判の良い学術誌の名前を真似たり、本人の同意なしに理事として学者を掲載したりするなど、欺瞞的な手法を用います。さらに、その手口はますます巧妙になり、正規のものと見分けるのが難しくなっています。しばしば本物そっくりのウェブサイトを開設し、表面的な査読を行うことで信憑性があるかのように装っています。そのため、研究者、特に資源に乏しい地域の研究者は、こうしたジャーナルに論文を投稿するよう惑わされてしまうのです。したがって、ハゲタカジャーナルを見分けるには、誤字脱字、連絡先のメールアドレスが不明瞭、信じがたいほど短い出版時期、高いアクセプト率、高額な一時金などの兆候を見極める鋭い目が必要です。
アカデミック・インテグリティの低下は、ハゲタカジャーナルから生じる重大な問題です。評判の良い学術誌に比べて査読期間が短く、リジェクト率も低いため、それを承知で出版する研究者もおり、質の低い研究の伝播につながっています。ありがたいことに、研究機関は研究者への支援を強化し、信頼できる学術誌で出版することの重要性を強調することで、ハゲタカジャーナルの影響と闘い始めています。要するに、ハゲタカジャーナルはより巧妙に進歩している中、科学研究と出版の完全性を守るためには、認識向上と研究機関の支援が不可欠なのです。
How to Identify Predatory Journals(2024.09)

2024.09.05 Editor T. J.
1. Verify the Journal's Evaluation and Credibility
- DOAJ (Directory of Open Access Journals): Lists reliable open access journals.
- JCR (Journal Citation Reports): Provides impact factors and other metrics to evaluate the quality of journals.
2. Thoroughly Examine the Journal's Website
- Contact Information: Check if there is a clear address and contact information. Be cautious if there are vague contact details or editorial staff use free or personal email domains.
- Editorial Board: Check the names and affiliations of the editorial board members and reviewers. If in doubt, contact these scholars directly to verify their involvement.
- Publication Time and Acceptance Rate: Be wary of journals that promise unusually short publication times or high acceptance rates.
3. Investigate the Journal's Reputation
- Beall’s List: Provides a list of predatory journals. Although not comprehensive, it is a useful reference.
- Researcher Networks and Forums: Verify the journal’s reputation with colleagues and within your field’s community. Experiences and opinions from other researchers can be valuable.
4. Check the Publishing Costs and Fees
- Upfront Fees: Be cautious of journals that demand high upfront fees or have unclear pricing structures. Reputable journals typically provide clear information about costs.
5. Assess the Quality of the Papers
- Published Papers: Read a few papers published in the journal to evaluate the quality of content and rigor of reviews. A high presence of low-quality papers is a red flag.
6. Verify Indexed Databases
- Databases like Scopus and PubMed: Check if the journal is indexed in major academic databases. Journals listed in these databases are generally more reliable.
7. Consult with Professional Institutions if in Doubt
- University or Research Institution Libraries: Seek advice on the journal’s credibility.
- Committee on Publication Ethics (COPE): Provides guidelines on publication ethics and can offer advice.
日本語訳は次へ↓
ハゲタカジャーナルの見分け方
1. ジャーナルの評価と信頼性を確認する
- DOAJ(Directory of Open Access Journals): 信頼できるオープンアクセスジャーナルのリスト。
- JCR(Journal Citation Reports): インパクトファクターなど、ジャーナルの質を評価するための指標を提供。
2. ジャーナルのウェブサイトを徹底的に調べる
- 連絡先: 住所や連絡先が明確かどうかを確認する。連絡先があいまいだったり、編集スタッフがフリーメールや個人のメールドメインを使っていたりする場合は要注意。
- 編集委員会 : 編集委員と査読者の氏名と所属を確認する。疑わしい場合は、これらの学者に直接連絡を取り、その関与を確認する。
- 出版時期と受理率: 異常に短い出版期間や高い受理率を約束しているジャーナルには要注意。
3. ジャーナルの評判を調べる
- Beallのリスト: ハゲタカジャーナルのリスト。包括的なものではないが、有用な参考資料。
- 研究者ネットワークとフォーラム: ジャーナルの評判を同僚や専門分野のコミュニティで確認する。他の研究者の経験や意見は重要。
4. 出版費用と料金の確認をする
- 初期費用: 高額な初期費用を要求するジャーナルや、料金体系が不明瞭なジャーナルには要注意。評判の良いジャーナルは通常、費用について明確な情報を提供している。
5. 論文の質を評価する
- 掲載論文: ジャーナルに掲載された論文をいくつか読み、内容の質とレビューの厳密さを評価する。質の低い論文が多い場合は、赤信号。
6. 索引付きデータベースの確認をする
- ScopusやPubMedなどのデータベース:ジャーナルが主要な学術データベースに索引付けされているかを確認。これらのデータベースに掲載されているジャーナルは、一般的に信頼性が高い。
7. 疑わしい場合は専門機関に相談する。
- 大学や研究機関の図書館: ジャーナルの信頼性について助言を求める。
- 出版倫理委員会(COPE): 出版倫理に関するガイドラインを提供し、助言を得ることができる。
Mastering Manuscripts: Essential Tips for Writing Your First Scientific Paper-Part1(2024.10)

2024.10.03 Editor T. J.
Mastering Manuscripts: Essential Tips for Writing Your First Scientific Paper
Writing your first scientific manuscript can be a daunting task, but understanding the essential do’s and don’ts can significantly improve the quality of your paper and increase its chances of being accepted for publication. This brief guide offers practical advice on what you should and shouldn’t do in each main section of your manuscript.
(1) Hook your readers with a captivating introduction
Do: Write a clear and concise introduction that provides necessary background, explains the rationale for your study, and states your research question or hypothesis. Aim for three to four short paragraphs that engage the reader and set the stage for your research.
Don’t: Overwhelm the reader with excessive background information. Keep your introduction focused and directly relevant to your research question. Avoid restating study findings in the final paragraph; this is repetitive and should be reserved for the abstract.
(2) Fill your methods with plenty of detail
Do: Provide a thorough description of your methods so that other researchers can replicate your study. Include details on materials, procedures, data collection, and analysis. Referring to your lab notebook can be a useful starting point.
Don’t: Omit crucial methodological details: Incomplete or vague descriptions can undermine the reliability and reproducibility of your results. Uniquely, you should not use a personal voice in this section – instead, use a passive, impersonal voice to maintain objectivity.
Example:
- “Proteins were analyzed by Western blotting” – Yes!
- “We analyzed protein expression by Western blotting” – No!
(3) Showcase your results with clarity and precision
Do: Organize your results logically, using tables, figures, and graphs to summarize key data. Use an active voice to engage readers with your discoveries.
Don’t: Include raw data or superfluous details. Focus on the results that are most pertinent to your research question and conclusions. Additional data can be placed in supplementary materials if necessary.
To be continued…
日本語訳は次へ↓
論文作成の極意:初めての科学論文執筆に役立つヒント
初めての科学論文の執筆は大変な作業ですが、重要な「すべきこと」と「すべきでないこと」を理解することで、論文の質が大幅に向上し、論文がアクセプトされる可能性が高まります。この簡潔なガイドでは、論文の各主要セクションで「すべきこと」と「すべきでないこと」について、実践的なアドバイスを提供しています。
(1) 魅力的な導入部分で読者を惹きつける
すべきこと:必要な背景情報を提供し、研究の根拠を説明し、研究の問いまたは仮説を提示する、明確で簡潔な導入部を書くことを心がけましょう。 3~4つの短い段落で、読者を引き込み、研究の舞台を整えることを目指しましょう。
すべきでないこと:背景情報を過剰に盛り込み、読者を圧倒しないようにしましょう。 導入部は焦点を絞り、研究の問いに直接関連する内容に留めましょう。 最終段落で研究結果を繰り返し述べないこと;これは冗長であり、要約に留めておくべきです。
(2) 方法の詳細を十分に記載する
すべきこと:他の研究者が研究を再現できるよう、方法について詳細に記述するよう心がけましょう。材料、手順、データ収集、分析の詳細を含めます。研究室のノートを参照すると、有益な出発点となるでしょう。
すべきでないこと: 重要な方法論の詳細を省略しないこと:不完全な記述や曖昧な記述は、結果の信頼性と再現性を損なう可能性があります。このセクションでは、主観的な表現は避け、客観性を維持するために、受動的で人称のない表現を心がけましょう。
例:
- 「タンパク質はウェスタンブロッティング法で分析された」 - はい!
- 「私たちはウェスタンブロッティング法でタンパク質の発現を分析した」 - いいえ!
(3) 結果を明確かつ正確に提示する
すべきこと:表、図、グラフを使用して論理的に結果を整理し、主要データを要約しましょう。発見について読者の関心を引くために能動態を使用しましょう。
すべきでないこと:生データや余計な詳細を含めないようにしましょう。研究の疑問や結論に最も関連する結果に焦点を置いてください。必要であれば、追加のデータを補足資料に記載することができます。
来月に続く・・・
Mastering Manuscripts: Essential Tips for Writing Your First Scientific Paper-Part2(2024.11)

2024.11.25 Editor T. J.
(4) Unpack the significance of your findings
Do: Interpret your results in the context of existing research. Discuss their significance, implications for the field, and any limitations of your study to demonstrate how analytical you can be.
Don’t: Overstate your conclusions or ignore the limitations of your study. Instead, you must be honest and balanced to maintain credibility and demonstrate your objectivity.
(5)Wrap it up with a strong conclusion
Do: Reinforce the key points of your research and offer new insights, such as potential applications or future research directions.
Don’t: Introduce new information or arguments in the conclusion. Keep the conclusion focused on summarizing and reflecting on your results.
(6)Take time to perfect and polish your submission package
Once your draft is complete, ensure you:
- Seek constructive feedback: Ask colleagues or mentors to review your manuscript. They might spot errors or offer different perspectives that enhance your paper.
- Proofread like a perfectionist: Carefully check for grammatical errors, typos, and inconsistencies. A well-edited manuscript facilitates smoother peer review and publication.
- Follow journal guidelines to the letter: Adhere to the formatting and style guidelines of your target journal, including those for figures and supplementary files. Attention to detail is crucial.
- Ensure clarity and flow: Use clear, concise language, avoid jargon, and ensure that your manuscript is well-organized with logical headings and flow.
- Stay objective: Avoid exaggerative terms like "revolutionary" or "groundbreaking" and use precise, evidence-based language to maintain objectivity and credibility in scientific writing. You can do so by referring to statistical significance wherever possible.
Summary
Writing a scientific manuscript is both complex and rewarding. By following these key do’s and don’ts, you can enhance the quality of your paper and improve its chances of publication. Remember, persistence and attention to detail are essential in scientific writing and with practice, you'll refine your skills and gain confidence.
日本語訳は次へ↓
論文作成の極意:初めての科学論文執筆に役立つヒント-Part2
(4) 調査結果の意義を明確にする
すべきこと:結果を既存の研究との関連で解釈しましょう。調査結果の意義、その分野への影響、研究の限界について論じ、分析能力の高さを示しましょう。
すべきでないこと:結論を誇張したり、研究の限界を無視したりしてはいけません。代わりに、信頼性を維持し、客観性を示すために、正直かつ公平であることが求められます。
(5) 結論を力強くまとめる
すべきこと:研究の主要なポイントを強調し、潜在的な応用や今後の研究の方向性など、新たな洞察を提供しましょう。
すべきでないこと:結論で新たな情報や論点を提示すること。結論では、結果の要約と考察に焦点を絞りましょう。
(6) 提出書類一式を完成させ、磨き上げるために時間をかけましょう
下書きが完成したら、次のことを確認してください。
- 建設的なフィードバックを求める:同僚や指導者に原稿の査読を依頼しましょう。原稿の誤りを指摘してもらえたり、論文をより良くする別の視点を提供してもらえるかもしれません。
- 完璧主義者のように校正する:文法上の誤り、誤字脱字、不統一がないか、入念にチェックしましょう。校正の行き届いた原稿は、査読や出版をよりスムーズに進めることができます。
- ジャーナルのガイドラインを厳密に守る:図表や補足ファイルも含め、投稿先のジャーナルのフォーマットやスタイルのガイドラインに従いましょう。細部への注意が重要です。
- 明瞭性と流れを確保する:明瞭で簡潔な表現を使い、専門用語は避け、論理的な見出しと流れで原稿がきちんと構成されていることを確認してください。
- 客観性を保つ:「革命的」や「画期的」といった誇張した表現は避け、科学的執筆における客観性と信頼性を維持するために、正確で根拠に基づいた表現を使用してください。可能な限り統計的有意性を参照することで、これを実現できます。
要約
科学論文の執筆は複雑ですが、やりがいのある仕事です。 これらの重要な「すべきこと」と「すべきでないこと」に従うことで、論文の質を高め、出版の可能性を高めることができます。 科学論文執筆には粘り強さと細部への注意が不可欠であることを忘れないでください。そして、練習を重ねることで、スキルを磨き、自信を深めることができます。
Greetings to the New Year(2024.12)

2024.12.10 Editor G. M.
Again, all too quickly, we find ourselves at the end of another year of, hopefully, very productive scientific and clinical work. Thanks to globalization, this is year has been marked by the continuation of inflationary pressures in many countries. While post-COVID inflation has subsided, this has been replaced by extreme weather events, a rise in energy costs and, for Japan, the depreciation of the yen that have resulted in a cost-of-living crisis that affects all aspects of the economy, including the ability to undertake medical research.
As an NAI editor of many years standing, I have come to appreciate the enormous amount of work authors engage in over, in some cases, many years before their endeavours reach the manuscript stage. Our authors all contribute greatly to their respective fields, ranging from reports on new immunotherapy combinations for various cancers in clinical trials to extensive review articles and unusual case studies. As editors, we are here to guide manuscripts along the tortuous path of submission and peer review through to successful publication. We hope to continue this important task for our highly esteemed and well-respected authors in 2025.
Wishing you all continued prosperity and happiness, both personal and professional, as we get ready to celebrate the coming of a New Year.
日本語訳は次へ↓
またしてもあっという間に、私たちは、科学と臨床の分野で非常に生産的な1年を終えようとしています。グローバル化のおかげで、今年は多くの国々でインフレ圧力が継続した年となりました。新型コロナウイルス感染症後のインフレは沈静化しましたが、それに代わって異常気象やエネルギーコストの上昇、そして日本では円安が起こり、医療研究の遂行能力を含む経済のあらゆる側面に影響を及ぼす生活費の高騰という危機をもたらしました。
長年NAIの編集に携わってきた私にとって、執筆者が原稿を完成させるまでに、場合によっては何年も前から膨大な作業を行っていることを理解しています。 私たちの執筆者は、臨床試験中のさまざまな癌に対する新しい免疫療法の組み合わせに関する報告から、広範なレビュー記事や珍しい症例研究まで、それぞれの分野に多大な貢献をしています。 編集者として、私たちは、原稿が投稿から査読を経て、最終的に出版されるまで、その曲がりくねった道筋を導いていく立場にあります。2025年にも、尊敬を集める著者の方々のために、この重要な任務を継続していきたいと考えています。
新年を迎えるにあたり、皆様のさらなるご繁栄とご多幸を心よりお祈り申し上げます。